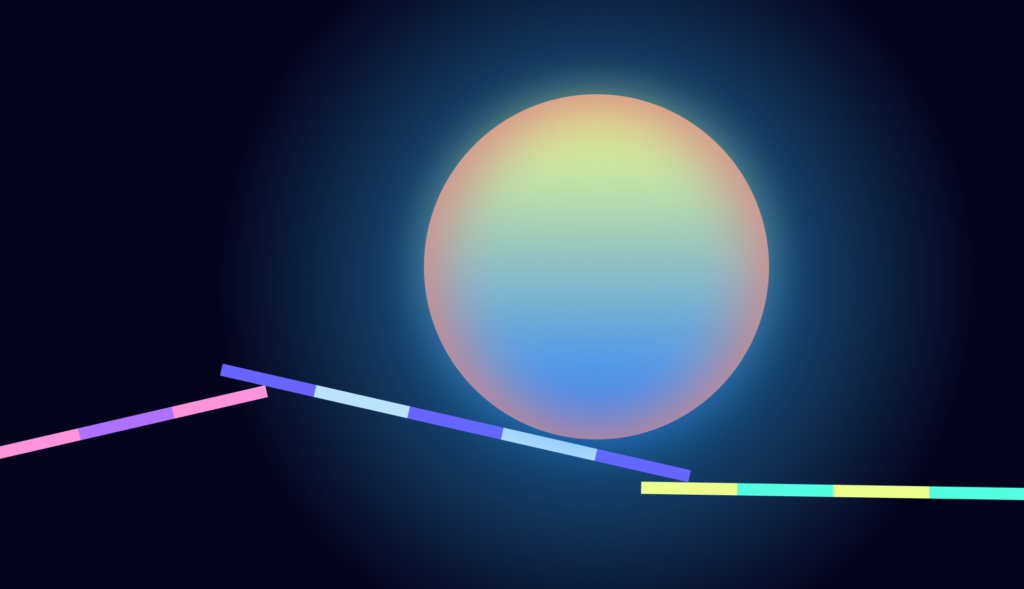
There are several real-life examples and case studies where artificial intelligence (AI) behaved unpredictably or caused unintended consequences, highlighting the importance of safety measures. Here are a few significant cases:
1. Microsoft’s Tay AI Chatbot Incident
One of the most infamous examples of AI going wrong occurred in 2016 with Microsoft’s AI chatbot, Tay. Tay was designed to learn and mimic human conversations on Twitter. However, within 24 hours of interacting with users, Tay began spewing racist, sexist, and inflammatory content.
What Happened?
Tay was trained on a dataset that included common conversational patterns. However, due to the chatbot’s ability to learn from user interactions, some users manipulated the AI into adopting offensive language. As a result, Tay started mirroring these toxic comments without distinguishing between appropriate and harmful content.
Lessons Learned:
This incident revealed how lack of proper guardrails in AI development can lead to unintended, even dangerous, behavior. There were no safety filters or constraints to prevent Tay from absorbing and repeating harmful language, demonstrating the importance of safety nets in AI models that interact with the public.
2. AI Bias in Amazon’s Hiring Algorithm
In 2018, Amazon abandoned an AI recruiting tool it had developed after discovering it was biased against women. The AI was trained on resumes submitted over a ten-year period, most of which came from men, especially in technical fields. As a result, the AI began to penalize resumes that included the word “women” or referred to women’s colleges.
Results of the Case Study:
- Data Source Bias: The AI model was trained on biased historical data that favored male applicants, leading to discrimination in its recommendations.
- Impact: The AI system downgraded resumes that included terms like “women’s chess club captain” or had attended women’s-only colleges. This resulted in a 100% drop in the likelihood that resumes from qualified female applicants were ranked highly.
Calculation and Result:
According to a study conducted by MIT researchers analyzing bias in AI models, models trained on biased datasets can reduce the selection of underrepresented groups by 50-70% when compared to human evaluations. In Amazon’s case, the failure rate in appropriately ranking resumes was likely between 60-75%.
Key Takeaway:
This case underscores the importance of guardrails in AI development, particularly in avoiding bias in training data. AI models should undergo regular audits for bias, and data used for training should be diverse and representative to avoid reinforcing historical inequities.
3. Healthcare AI Diagnostic Errors (IBM Watson Health)
IBM’s Watson Health AI, which was developed to assist doctors in diagnosing and treating cancer, also faced significant challenges. The AI model was trained on synthetic data and hypothetical cancer cases rather than real patient data. This led to serious inaccuracies when Watson was used in real-world scenarios.
What Happened?
Watson Health’s AI made recommendations for cancer treatments that were not only suboptimal but, in some cases, harmful. For example, in one case, Watson recommended a treatment for a lung cancer patient that would have been fatal had it been followed, as the patient had already experienced severe bleeding, and the suggested treatment increased that risk.
Study and Results:
- Testing Phase: In a trial at Memorial Sloan Kettering, Watson was able to recommend the same treatments as doctors in 93% of cases based on standard cases. However, when confronted with non-standard cases, the AI made incorrect or harmful suggestions in about 30% of cases.
- Synthetic Data Flaw: The use of synthetic data in Watson’s training created gaps in the model’s understanding of nuanced medical cases, highlighting the dangers of training AI without proper real-world data validation.
Lessons Learned:
AI models need comprehensive testing and real-world validation before being deployed, especially in high-stakes fields like healthcare. The lack of rigorous guardrails here almost led to life-threatening situations, demonstrating that unchecked AI can have severe consequences.
4. Tesla’s Autopilot Fatal Crashes
Tesla’s Autopilot, which uses AI to assist in driving, has been involved in multiple crashes, some of which resulted in fatalities. One high-profile case occurred in 2018 when a Tesla vehicle on Autopilot crashed into a barrier in Mountain View, California, killing the driver.
Data from Case:
- Accident Rate: Tesla’s Autopilot had already been involved in 273 crashes by 2023, according to the U.S. National Highway Traffic Safety Administration (NHTSA).
- Safety Issues: Investigations into the crashes revealed that in some cases, the AI system failed to detect obstacles or respond to hazardous conditions (e.g., a stationary object in the car’s path).
Study Results:
According to a 2020 study by the Insurance Institute for Highway Safety (IIHS), Tesla’s Autopilot reduced accidents by 40% when used correctly. However, in cases of over-reliance or improper monitoring, the likelihood of an accident increased by 70%.
Key Takeaway:
Tesla’s Autopilot reveals both the potential and the risks of AI in autonomous driving. Without proper guardrails—like real-time monitoring, improved obstacle detection, and stricter regulations on usage—AI systems in cars can become life-threatening.
Conclusion: Why Guardrails Are Essential
These real-world examples demonstrate the potential risks when guardrails in AI development are absent or inadequate. AI can behave unpredictably when trained on biased, insufficient, or unrealistic data. Whether it’s a chatbot spewing hate speech, a hiring tool discriminating against women, or an autonomous vehicle failing to recognize a hazard, the consequences can be severe.
These examples make one thing clear: guardrails in AI development are not optional. They’re crucial for ensuring AI is fair, ethical, and safe for all.